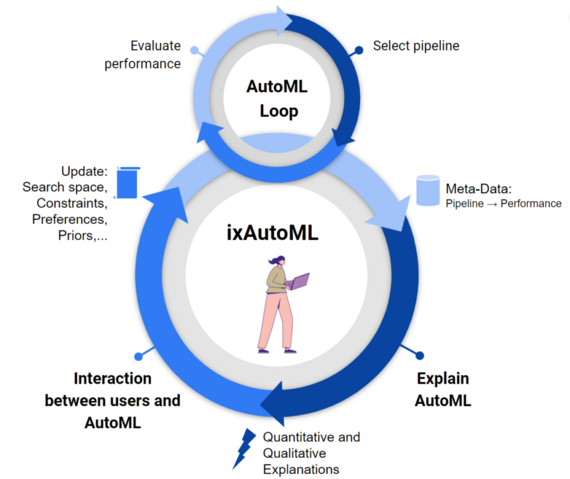
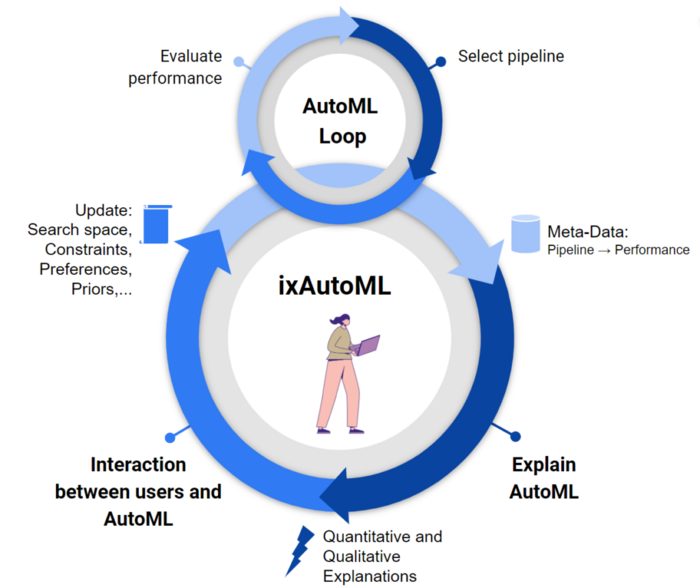
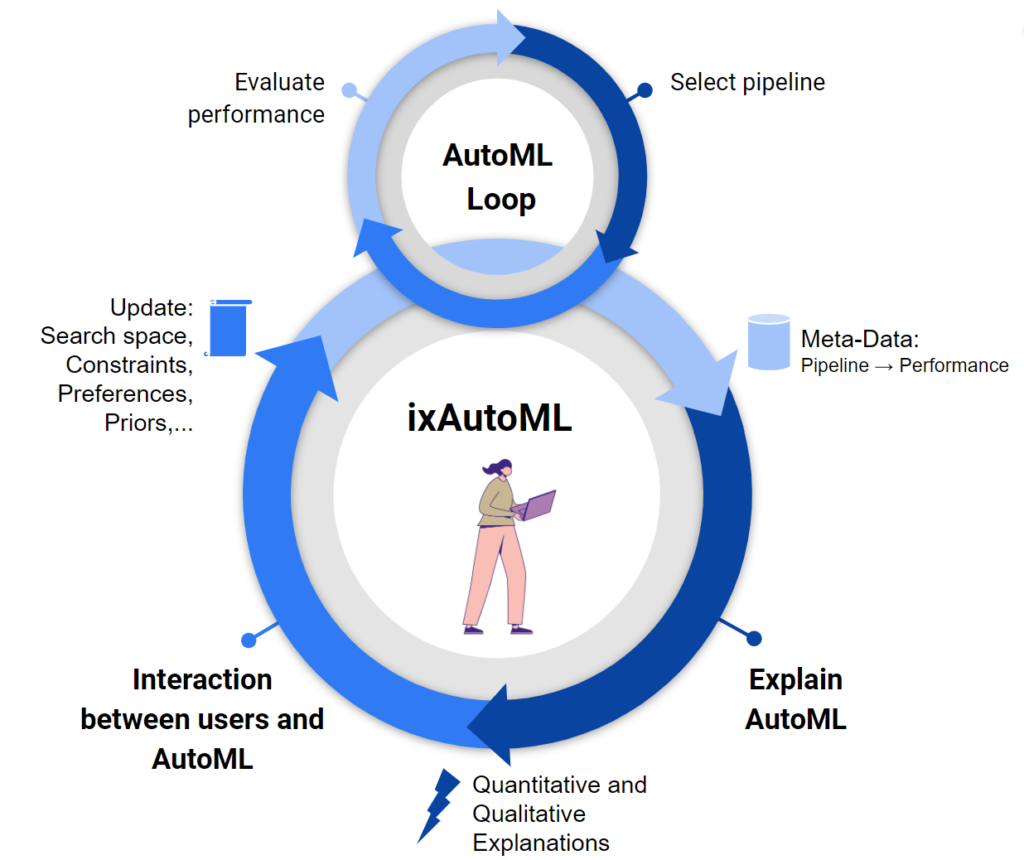
Funding Agency
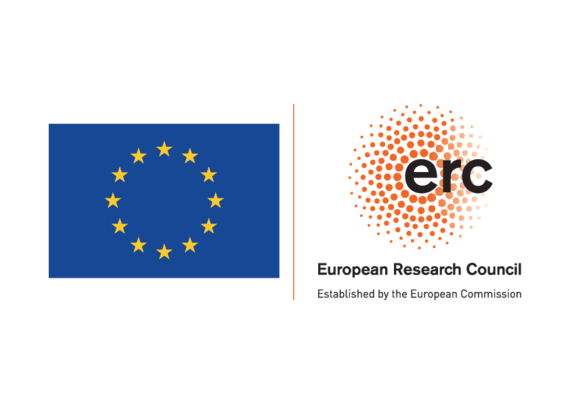
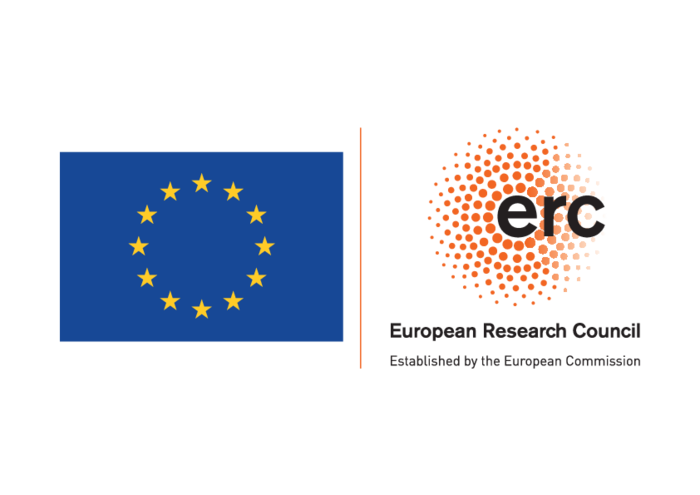
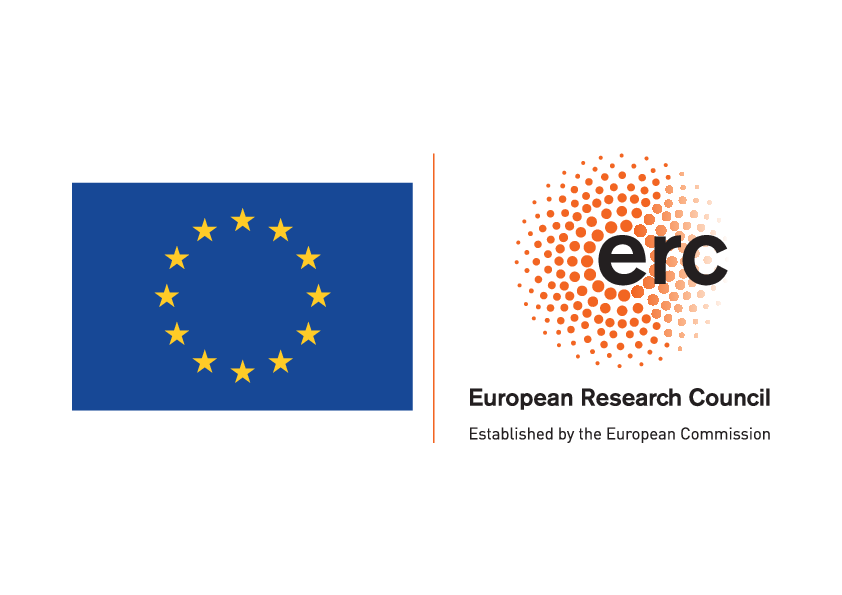
Trust and interactivity are key factors in the future development and use of automated machine learning (AutoML), supporting developers and researchers in determining powerful task-specific machine learning pipelines, including pre-processing, predictive algorithm, their hyperparameters and -if applicable- the architecture design of deep neural networks. Although AutoML is ready for its prime time, democratization of machine learning via AutoML is still not achieved. In contrast to previously purely automation-centered approaches, ixAutoML is designed with human users at its heart in several stages. The foundation of trustful use of AutoML will be based on explanations of its results and processes. To this end, we aim for: (i) Explaining static effects of design decisions in ML pipelines optimized by state-of-the-art AutoML systems. (ii) Explaining dynamic AutoML policies for temporal aspects of dynamically adapted hyperparameters while ML models are trained. These explanations will be the base for allowing interactions allowing to combine human intuition and generalization capabilities for complex systems, and efficiency of systematic optimization approaches for AutoML.
Lead at LUHAI: Prof. Lindauer
Funding Program: ERC Starting Grant
Project Period: Dec 2022 - Nov 2027
Publications
Zeige Ergebnisse 1 - 7 von 7